Reliability, Validity, and Accuracy of Experiments
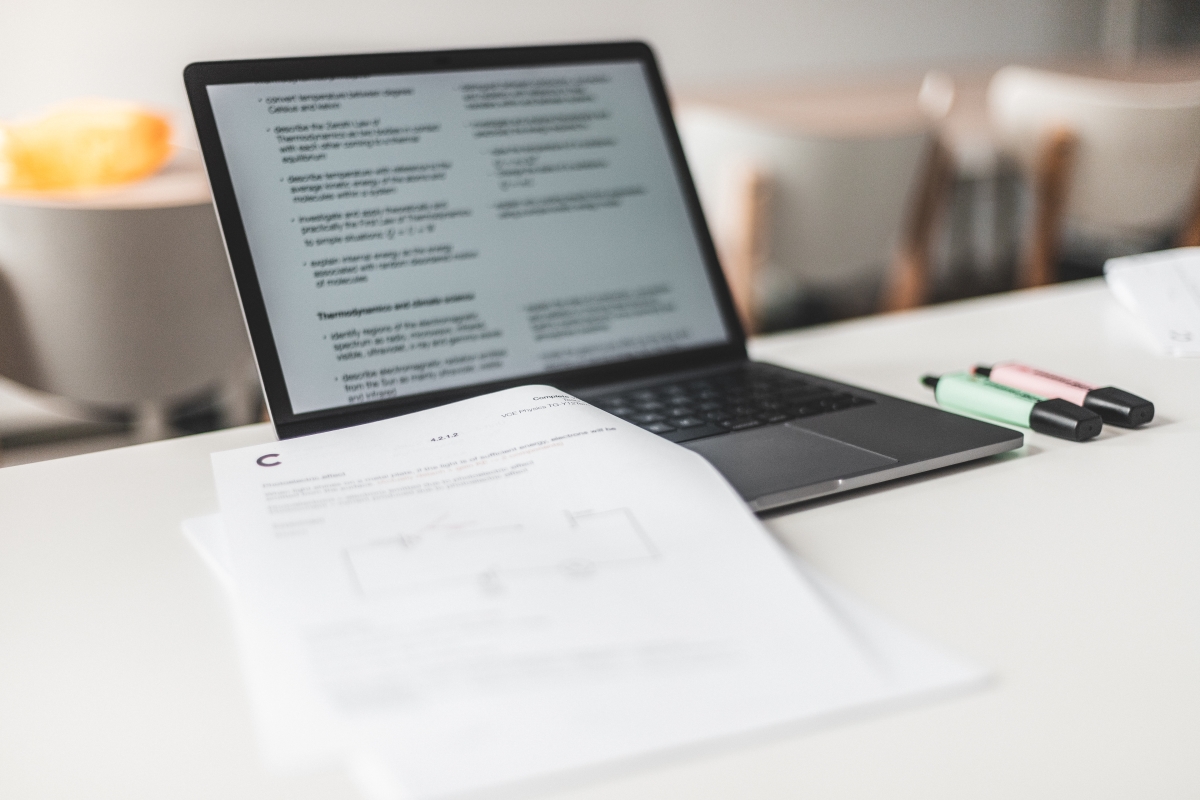
Introduction
In your VCE Physics practical, you will be tested on your ability to conduct experiments and analyse results. To do so, it is important to understand the concepts of validity, reliability, accuracy, and precision .
Each term has a distinct definition, though they are often conflated or confused with one another. Let us clarify them so you can better design experiments, analyze results, and judge the soundness of findings.
Summary
Validity refers specifically to whether an experiment measures what it purports to measure. For example, a test designed to assess math skills would have high validity if it focuses only on math questions and not unrelated subject areas. Validity ensures that experimental results reflect the phenomenon under study rather than extraneous variables.
Reliability describes the consistency and replicability of research findings and experimental outcomes. A reliable experimental protocol will yield the same results time after time when repeated by multiple researchers. Reliability provides assurance that results are not due to random chance.
Accuracy concerns how close experimental measurements or data are to the true or accepted value. High accuracy means that any errors are minimal and small in magnitude. Improving accuracy involves reducing both random error and systematic biases.
Precision relates to the level of detail or resolution in an experimental measurement. For instance, a scale that measures weight to the nearest tenth of a gram has higher precision than one that measures only whole grams. Precision is independent from accuracy.
What is Validity?
Validity focuses on whether an experiment actually measures what it is intended to measure. It ensures that the results obtained from the experiment are meaningful and significant.
In other words “Does my experiment actually measure what I intent to in the aim”?
Internal Validity
Internal validity looks at whether the cause and effect relationship between the independent and dependent variables is a strong one. In an experiment we are investigating whether X (independent variable) causes Y (dependent variable), all other variables being equal (control variables). Confounding variables that provide alternative explanations for results must be controlled.
External Validity
External validity concerns whether results can be generalized beyond the specific study conditions and sample group. The participants, environment and methods should represent the broader population and real-world situations.
How to Test & Improve it?
To ensure high validity, you must:
- Clearly define variables and constructs being measured
- Demonstrate logical relationships between measures used and outcomes
- Control confounding variables
- Replicate studies under different conditions with different samples
- Check that the test or experiment actually measures the intended concept
- Address & address assumptions
Following strong methodology and research design principles enhances validity. Validity ensures the results are robust and meaningful.
What is Reliability?
Reliability refers to the consistency of measurements or experiment outcomes when repeated under the same conditions. It helps determine whether results are reproducible and not due to chance.
There are several types of reliability:
Test-Retest Reliability
This involves administering the same test twice over a period of time to the same group of individuals. High test-retest reliability means the scores are similar between the two tests. This helps evaluate the stability of results over time.
Inter-Rater Reliability
This evaluates the degree to which different raters or observers give consistent estimates or ratings of the same behavior or phenomenon. High inter-rater reliability indicates the ratings are consistent across raters.
How to Test & Improve it?
To ensure high reliability:
- Use standardized instructions and procedures for administering tests or experiments. This reduces variability.
- Ensure observers and raters are properly trained so ratings are calibrated across people.
- Increase sample sizes, which reduces the variability of mean values between tests.
- Carefully design experiments and tests to isolate the effect and relationship you want to measure.
Reliability is essential for valid interpretation of results. Methods and metrics with high reliability give researchers confidence the results reflect true effects rather than random fluctuations.
What is Accuracy?
Accuracy refers to how close the results of a measurement or experiment are to the true or accepted value. It is different from validity, which focuses on whether the experiment actually measures what it is intended to measure. Accuracy is also separate from reliability, which refers to consistency of results when measurements are repeated.
While high validity and reliability are important, a measurement can be valid and reliable but inaccurate. For example, a scale may consistently report someone's weight as 10 pounds higher than their true weight. The measurement would have high reliability but low accuracy.
How to Test & Improve it?
There are a few key ways to improve accuracy in experiments and measurements:
- Use equipment and methods that are known to produce accurate, error-free results when used properly. For example, use a properly calibrated scale rather than one that constantly misreports values.
- Take multiple measurements and use statistical techniques to minimize random errors. Averaging multiple measurements reduces the effect of random fluctuations.
- Identify and minimize systematic errors - errors that shift all measurements consistently higher or lower than the true value. This may involve adjustments like accounting for equipment calibration drift.
- Use the most precise measurement tools available to capture the highest level of detail. This avoids rounding or estimation errors.
- Ensure the experiment and equipment used are appropriate for measuring the desired variable, rather than inadvertently measuring something else. This improves accuracy by measuring the intended target.
Paying attention to accuracy, in addition to validity and reliability, helps ensure research results closely reflect the true, accepted values in the real world. Accurate measurements instill greater confidence in the conclusions drawn from an experiment.
What is Precision?
What is it?
Precision refers to the level of detail in measurements. It is determined by the unit of measurement being used. For example, a measurement of 5 meters is less precise than one of 5.2 meters. Precision relates to reproducibility - how close repeated measurements are to each other.
Precision is different from accuracy. A measurement can be extremely precise but not accurate. For example, if a scale consistently measures an object at 5.00000 kg but its true weight is 5.2 kg, the measurement is very precise but not accurate.
How to Test & Improve it?
Precision depends on the instrumentation being used and the care taken when recording measurements. Ways to increase precision include:
- Using instruments capable of measuring in smaller units, like millimeters instead of centimeters. This provides more decimal places and detail.
- Making multiple measurements and reporting results to a consistent number of decimal places. Don't round early in calculations.
- Using the proper techniques when taking measurements and reading instruments to get consistent, repeatable results.
- Calibrating instruments regularly to manufacturer specifications.
- Reporting measurements with clear units and to an appropriate number of significant figures based on the precision of the instrument.
While accuracy is about trueness to the real value, precision relates to the level of reproducibility and detail in measurements. Increasing instrumentation precision allows more definitive comparisons between measurements and minimizes uncertainty.
What is Systematic Errors?
Systematic errors, also known as experimental bias, are errors that consistently occur due to inaccuracies in the equipment or methodology used in an experiment. Unlike random errors, systematic errors follow identifiable patterns or trends that influence the results in one direction. They affect the accuracy of a measurement by shifting all the results in a systematic way.
Some examples of systematic errors include:
- Instrument calibration errors: When the equipment used to take measurements is not properly calibrated, it can consistently give inaccurate readings. For example, a miscalibrated analytical balance may overestimate or underestimate the true mass of a sample.
- Observer bias: If the person conducting the experiment unintentionally influences the results in one direction, this is a systematic error. They may inadvertently affect the observation or recording of data based on subjective expectations.
- Environmental factors: Changes in environmental conditions like temperature or humidity can cause systematic deviations if they are not controlled for. For example, increased ambient temperature could expand the scale being used to take measurements.
- Instrument drift: If the performance of equipment deteriorates over time, such as a sensor becoming less sensitive, this can induce a directional bias in the measurements.
- Sampling errors: Taking non-random samples or disproportionate samples can skew results in a particular direction away from the true population value.
Systematic errors reduces Accuracy
The effect of systematic errors is an overall shift in the accuracy of results that leads to consistently over- or underestimated values. Unlike random errors which can cancel out, systematic errors accumulate and affect the accuracy in a defined direction. Identifying and minimizing sources of systematic error is crucial for improving the accuracy of experimental results. Careful calibration, eliminating observer bias, controlling environmental factors, and random sampling help reduce systematic errors.
What is Random Errors?
Random errors, also known as random variation, refer to inaccuracies that occur unpredictably and inconsistently in measurements. They are caused by factors that vary randomly between measurements, making the results scatter randomly above or below the true value.
Random errors cannot be corrected as their causes are unknown. However, they can be reduced by taking multiple measurements and calculating an average. The more measurements that are taken, the lower the random error. Taking an average also has a smoothing effect that cancels out the random fluctuation in readings.
There are several common causes of random errors:
- Small irregularities in instruments that affect each measurement differently
- Tiny environmental changes like temperature fluctuations
- Imperfections in experimental techniques between trials
- Biological variance when measuring living subjects
For example, when using a scale to weigh an object multiple times, the readings may be 4.2 lbs, 4.1 lbs, 4.3 lbs, 4.25 lbs due to slight variations in the instrument, air currents, and handling between each measurement.
Random errors reduces Reliability & Precision
Random errors reduce reliability and precision. But they do not affect accuracy systematically because the variation is symmetric around the true value. Reducing random errors improves the repeatability and reproducibility of experimental results. Careful experimental design is key, by controlling variables, standardizing procedures, calibrating equipment, and averaging repeated measurements.
Conclusion
In summary, validity, reliability, accuracy, and precision are important concepts in experimental design and measurement. Though often used interchangeably, there are key differences between these terms:
- Validity refers to whether an experiment measures what it is intended to measure. Validity can be improved through careful selection of appropriate equipment and controls.
- Reliability relates to the consistency of results when measurements are repeated under identical conditions. Reliability can be enhanced by minimizing random errors in the experimental procedure.
- Accuracy refers to how close a measurement is to the true value. Accuracy is affected by systematic errors which can be minimized through proper calibration and technique.
- Precision relates to the exactness of measurements and is expressed through the number of significant figures. Precision is not the same as accuracy.
Carefully considering and optimizing for validity and reliability in experimental design is crucial for generating meaningful results. Valid, reliable data allows researchers to draw accurate conclusions from their work. Paying attention to these factors demonstrates scientific rigor and helps advance knowledge within a field.